Hello! I am an Engineer at Sprinklr AI, in the Conversational AI team developing FAQ and Smart Recommendation bots.
My interests lie in how we can utilize large-scale foundation models to enable intuitive human-robot interaction.
Previously, I graduated from IIIT-Hyderabad with a Dual (B.Tech + MS) Degree in Computer Science, where I studied autonomous driving and swarm robots at the Robotics Research Center under Prof. Madhava Krishna and Prof. Harikumar Kandath, and published papers at conferences like IEEE ICRA, CASE and ICARA.
If you wish to connect, please drop an email to vikrant.dewangan@research.iiit.ac.in
News
Dec, 2024 | Our paper “When Every Token Counts” on tokenization for Low-Resource languages is accepted into “LoResLM @ COLING 2025” ! |
Mar, 2024 | Served as a reviewer for IROS-2024 |
Jan, 2024 | Our paper on Vision-language models in Autonomous Driving titled “Talk2BEV: Language-enhanced Bird’s Eye View maps” gets accepted into ICRA-2024 |
Dec, 2023 | Defended my Master’s thesis at IIIT-Hyderabad. |
Nov, 2023 | Our paper on Swarm Robotics titled “MPC-Based Obstacle Aware Multi-UAV Formation Control Under Imperfect Communication” gets accepted into ICARA-2024 |
Oct, 2023 | Served as a reviewer for ICRA-2024 and IEEE ICGVIP-2024 |
May, 2023 | Our paper on Uncertainty titled UAP-BEV: Uncertainty Aware Planning in Bird’s Eye View Representations accepted into CASE-2024 |
2022 | Joined Robotics Research Center as a researcher |
2018 | Started at IIIT Hyderabad as an undergraduate student |
Selected Publications
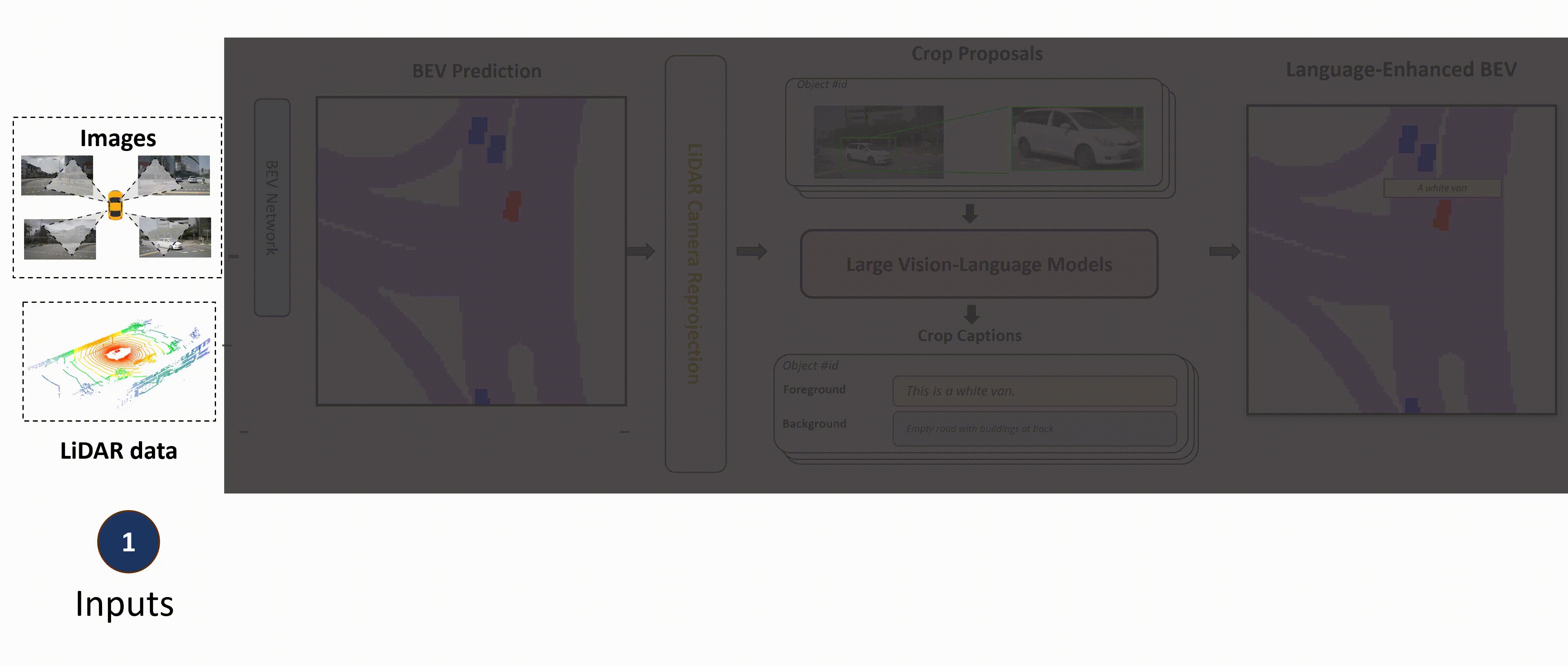
Talk2BEV: Language-Enhanced Bird's Eye View (BEV) Maps
ICRA 2024
Talk2BEV is a large vision-language model (LVLM) interface for bird's-eye view (BEV) maps in autonomous driving contexts. While existing perception systems for autonomous driving scenarios have largely focused on a pre-defined (closed) set of object categories and driving scenarios, Talk2BEV blends recent advances in general-purpose language and vision models with BEV-structured map representations, eliminating the need for task-specific models. This enables a single system to cater to a variety of autonomous driving tasks encompassing visual and spatial reasoning, predicting the intents of traffic actors, and decision-making based on visual cues. We extensively evaluate Talk2BEV on a large number of scene understanding tasks that rely on both the ability to interpret free-form natural language queries, and in grounding these queries to the visual context embedded into the language-enhanced BEV map. To enable further research in LVLMs for autonomous driving scenarios, we develop and release Talk2BEV-Bench, a benchmark encompassing 1000 human-annotated BEV scenarios, with more than 20,000 questions and ground-truth responses from the NuScenes dataset.
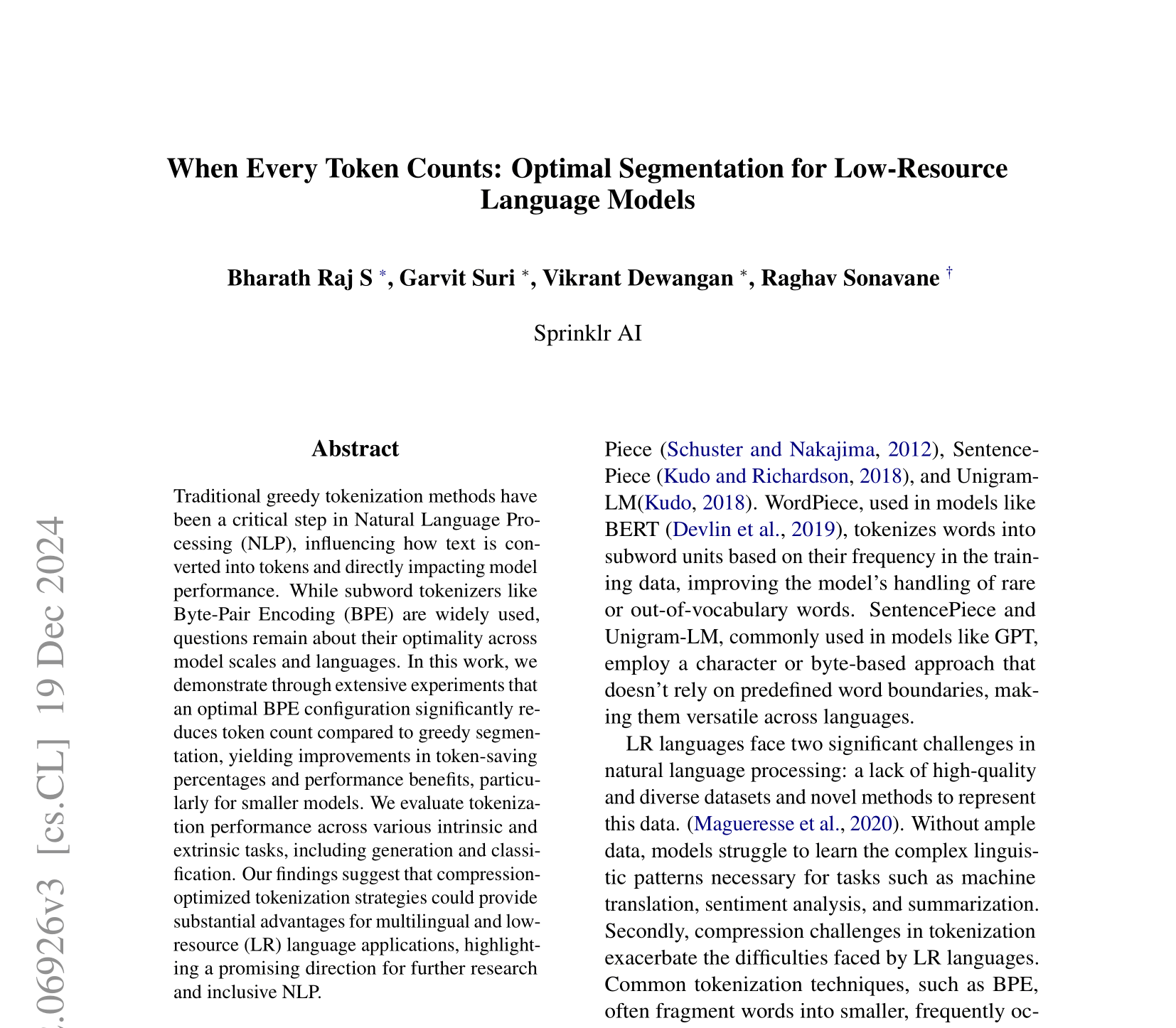
When Every Token Counts: Optimal Segmentation for Low-Resource Language Models
Language Models for Low-Resource Languages (LoResLM) Workshop @ COLING 2025
Traditional greedy tokenization methods have been a critical step in Natural Language Processing (NLP), influencing how text is converted into tokens and directly impacting model performance. While subword tokenizers like Byte-Pair Encoding (BPE) are widely used, questions remain about their optimality across model scales and languages. In this work, we demonstrate through extensive experiments that an optimal BPE configuration significantly reduces token count compared to greedy segmentation, yielding improvements in token-saving percentages and performance benefits, particularly for smaller models. We evaluate tokenization performance across various intrinsic and extrinsic tasks, including generation and classification. Our findings suggest that compression-optimized tokenization strategies could provide substantial advantages for multilingual and low-resource language applications, highlighting a promising direction for further research and inclusive NLP.